Intro
Unlock data insights with 5 essential Data Science Templates, leveraging machine learning, predictive analytics, and data visualization to drive business decisions and boost productivity.
Data science has become an integral part of various industries, including healthcare, finance, and technology. With the increasing amount of data being generated every day, the need for efficient data analysis and interpretation has never been more pressing. Data science templates can help streamline the process of data analysis, making it easier for data scientists and analysts to extract insights and make informed decisions. In this article, we will explore five data science templates that can be used to improve data analysis and interpretation.
The importance of data science templates cannot be overstated. They provide a structured approach to data analysis, ensuring that all necessary steps are taken to extract meaningful insights from data. By using data science templates, data scientists and analysts can save time and effort, reduce errors, and improve the overall quality of their analysis. Moreover, data science templates can be customized to suit specific needs and requirements, making them a versatile tool for data analysis.
Data science templates can be applied to various industries and domains, including business, healthcare, and finance. For instance, in business, data science templates can be used to analyze customer behavior, predict sales trends, and identify areas of improvement. In healthcare, data science templates can be used to analyze patient data, identify high-risk patients, and develop personalized treatment plans. The applications of data science templates are numerous, and their use can have a significant impact on the quality and efficiency of data analysis.
Introduction to Data Science Templates

Benefits of Data Science Templates
The benefits of data science templates are numerous. Some of the key benefits include: * Improved efficiency: Data science templates can help streamline the process of data analysis, reducing the time and effort required to extract insights from data. * Increased accuracy: Data science templates can help reduce errors and improve the overall quality of data analysis. * Enhanced collaboration: Data science templates can be shared and collaborated on by multiple stakeholders, improving communication and reducing misunderstandings. * Customizability: Data science templates can be customized to suit specific needs and requirements, making them a versatile tool for data analysis.Template 1: Data Exploration Template

Steps Involved in Data Exploration
The steps involved in data exploration include: 1. Data cleaning and preprocessing: This involves handling missing values, removing duplicates, and transforming variables. 2. Data visualization: This involves creating plots and charts to visualize the data and identify patterns and trends. 3. Summary statistics and data description: This involves calculating summary statistics, such as means and standard deviations, and describing the data in terms of its distribution and central tendency. 4. Correlation analysis and feature selection: This involves analyzing the relationships between variables and selecting the most relevant features for further analysis.Template 2: Predictive Modeling Template

Steps Involved in Predictive Modeling
The steps involved in predictive modeling include: 1. Data splitting and preprocessing: This involves splitting the data into training and testing sets and preprocessing the data for modeling. 2. Model selection and training: This involves selecting a suitable model and training it on the training data. 3. Model evaluation and hyperparameter tuning: This involves evaluating the model on the testing data and tuning its hyperparameters to improve its performance. 4. Model deployment and monitoring: This involves deploying the model in a production environment and monitoring its performance over time.Template 3: Data Visualization Template
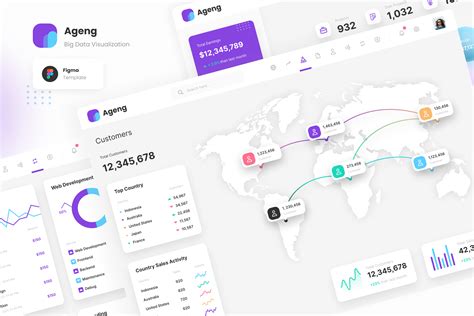
Steps Involved in Data Visualization
The steps involved in data visualization include: 1. Data selection and preprocessing: This involves selecting the relevant data and preprocessing it for visualization. 2. Visualization selection and creation: This involves selecting a suitable visualization type and creating it using a visualization tool. 3. Visualization customization and refinement: This involves customizing and refining the visualization to improve its appearance and effectiveness. 4. Visualization deployment and sharing: This involves deploying the visualization in a production environment and sharing it with stakeholders.Template 4: Machine Learning Template

Steps Involved in Machine Learning
The steps involved in machine learning include: 1. Data preprocessing and feature engineering: This involves preprocessing the data and engineering features to improve the model's performance. 2. Model selection and training: This involves selecting a suitable model and training it on the training data. 3. Model evaluation and hyperparameter tuning: This involves evaluating the model on the testing data and tuning its hyperparameters to improve its performance. 4. Model deployment and monitoring: This involves deploying the model in a production environment and monitoring its performance over time.Template 5: Deep Learning Template

Steps Involved in Deep Learning
The steps involved in deep learning include: 1. Data preprocessing and feature engineering: This involves preprocessing the data and engineering features to improve the model's performance. 2. Model selection and training: This involves selecting a suitable model and training it on the training data. 3. Model evaluation and hyperparameter tuning: This involves evaluating the model on the testing data and tuning its hyperparameters to improve its performance. 4. Model deployment and monitoring: This involves deploying the model in a production environment and monitoring its performance over time.Data Science Templates Image Gallery

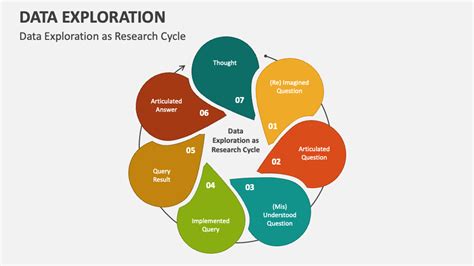





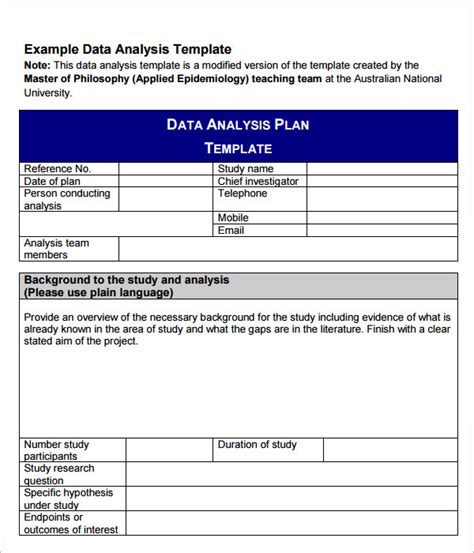


What are data science templates?
+Data science templates are pre-designed frameworks that provide a structured approach to data analysis. They typically include a series of steps and tasks that need to be completed to extract insights from data.
What are the benefits of using data science templates?
+The benefits of using data science templates include improved efficiency, increased accuracy, enhanced collaboration, and customizability. Data science templates can help streamline the process of data analysis, reduce errors, and improve the overall quality of data analysis.
How can I use data science templates in my organization?
+You can use data science templates in your organization by identifying the specific needs and requirements of your project, selecting a suitable template, and customizing it to suit your needs. You can also share and collaborate on data science templates with multiple stakeholders to improve communication and reduce misunderstandings.
What are some common types of data science templates?
+Some common types of data science templates include data exploration templates, predictive modeling templates, data visualization templates, machine learning templates, and deep learning templates. These templates can be used for various types of data analysis, including exploratory data analysis, predictive modeling, and data visualization.
How can I create my own data science template?
+You can create your own data science template by identifying the specific needs and requirements of your project, selecting a suitable template, and customizing it to suit your needs. You can also use a template as a starting point and modify it to create a new template that suits your specific needs.
In conclusion, data science templates are a valuable tool for data scientists and analysts. They provide a structured approach to data analysis, ensuring that all necessary steps are taken to extract meaningful insights from data. By using data science templates, data scientists and analysts can save time and effort, reduce errors, and improve the overall quality of their analysis. We encourage you to try out these templates and explore the many benefits they have to offer. Share your experiences and feedback with us, and don't hesitate to reach out if you have any questions or need further assistance.